An AI Model to Detect Damages on Historic Heritage Walls
Built heritage and traditional dwellings, is a testimony to the history and culture of an area. However, in areas such as Zhejiang Province, China, a humid climate and harsh weather conditions challenge the integrity of these buildings, causing significant damage such as cracks, holes, stains and yellowing of the wall surfaces. This damage requires timely interventions to maintain the integrity and aesthetics of the structures, prolonging their useful life.
Protecting this widespread heritage faces significant challenges. Rural villages are often scattered, making the manual inspection of the numerous walls a slow, expensive and exhausting process. High-tech measurement technologies, such as 3D laser scanners or ultrasound diagnostics, are expensive to acquire and operate and can have errors in accuracy. A more efficient and accessible approach is needed.
This is where digital technology and artificial intelligence come into play. A recent study by Shuai Yang and colleagues, published in npj Heritage Science, proposes and verifies an innovative method for the automatic identification of damage on brick walls of traditional villages in Zhejiang, using the deep learning model YOLOv8.
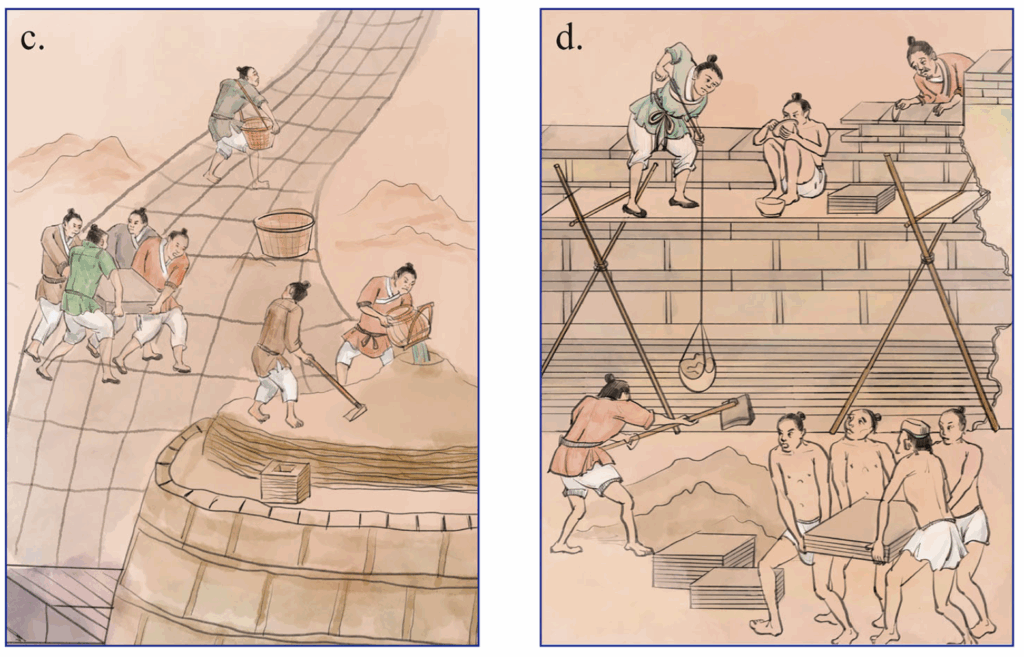
The Study and the Method
The researchers focused on traditional dwellings in the cities of Hangzhou and Lishui, characterized by a humid subtropical monsoon climate that contributes to the degradation of local building materials, such as red clay bricks. The most common damage identified was classified into four types: holes, white spots, yellowing (or browning) and cracks. The causes of this damage are varied, linked both to environmental factors (erosion, humidity, temperature changes, salts in the soil) and to traditional construction techniques and local materials (filled hollow walls, primitive brick kilns, loosening mortar).
To train the AI model, the researchers collected 196 high-resolution images of brick samples. After a selection and cropping process, they obtained 187 valid samples of size 512 × 512 pixels, covering the four types of damage. This dataset was divided into training (70%, about 131 images), validation (20%) and test (10%) sets, using data enhancement techniques (such as rotations and flips) to increase the robustness of the model.
The YOLOv8 model was chosen as the core of the system. Its architecture is based on multi-level feature extraction and fusion to improve detection, even for small damage or hidden areas.
The research process included data collection and processing, accurate damage labeling in the images (defining bounding boxes for each damage), training the model through 200 epochs, evaluating its performance with metrics such as average precision (AP), F1 score, recall and accuracy, and finally practical application via a mobile device.
Results and Practical Application
Tests showed that the model achieved promising results in damage detection. In particular, the model trained for 100 epochs demonstrated an optimal balance in performance across different damage types, with high accuracy for yellowing (AP 0.8, F1 0.71) and good recognition for spots (F1 score 0.71, accuracy close to 0.56). The ability to detect yellowing is particularly relevant, as it is closely linked to the effects of high humidity and salt migration in bricks.
The study also revealed limitations: crack detection remained low (accuracy almost 0 in many tests), and omissions in the detection of small holes were found. These aspects require further optimization.
A highlight of the study is the development of a mobile real-time detection device based on a device equipped with a camera and a servo system to adjust the angle, can be controlled via WiFi from a smartphone.
Field tests, conducted on real historical buildings, demonstrated its practicality and robustness in complex outdoor environments and its effectiveness in identifying damage with high-resolution images
Importance for Cultural Heritage Management
This study marks a significant step in the application of artificial intelligence for the protection of rural cultural heritage. The introduction of an automatic damage detection method offers several crucial advantages for heritage management:
- Efficiency and Cost Reduction: By automating the wall inspection process, the study proposes a solution that overcomes the cost and time limitations of manual inspection or other advanced technologies. This is crucial for large-scale protection in dispersed rural areas.
- Proactive Monitoring: The ability to quickly detect and locate damage enables timely repair interventions. Identifying problems such as cracks or stains in the early stages can prevent greater and more costly deterioration in the future.
- Informed Decision Support: The creation of a standardized database of damage annotations and the data collected via the mobile device provide systematic information for maintenance and restoration decisions, improving the scientificity and sustainability of interventions.
- Accessibility of Technology: The use of low-cost platforms such as Raspberry Pi 5 makes this technology potentially accessible even for local communities or small institutions with limited resources.
- New Perspectives for Conservation: The study provides a new perspective and a solid technological support for the long-term conservation of traditional dwellings, demonstrating how AI can be a crucial tool to safeguard and enhance rural built heritage.
Although challenges remain, particularly in the detection of very subtle damage such as hairline cracks, this work demonstrates the transformative potential of artificial intelligence in the field of cultural heritage conservation. The aim is to extend this technology to cover a wider range of materials and structures, transforming inspection and maintenance from manual and reactive processes to an intelligent, efficient and proactive system. In an era of rapid digitalization, the integration of AI in the management of rural heritage is a fundamental step to ensure its survival and transmission to future generations.
Final Considerations and Development Perspectives
Despite the promising results, it is essential to underline that the model has been trained on a relatively limited number of samples (187 images), which represents a significant constraint in terms of generalization and robustness of the system. This aspect highlights the need for further development and testing phases, which include:
- Expansion of the dataset: collecting images from a greater number of buildings, geographical areas and environmental conditions, to increase the representativeness and diversity of the data.
- Improvement of the detection of more complex damages: such as micro-cracks, currently difficult to identify by the model.
- Validation in real and diversified operational scenarios, to test the system’s ability to adapt to various architectural and environmental contexts.
Only through continuous methodological refinement and a broader database will it be possible to make this technology a fully reliable and replicable tool for the protection of widespread cultural heritage.